Reinforcement Learning - Steered Molecular Dynamics (RL-SMD)
A novel method that integrates reinforcement learning with robotics planning to chart low-energy molecular transition pathways, employing enhanced sampling techniques.
-Development of a framework combining reinforcement learning with robotics planning for molecular dynamics analysis
-Application of Jarzynski’s equality and stiff-spring approximation for accurate energy estimations at the atomic level
-Implementation of policy-driven adaptive steered molecular dynamics (SMD), a first in this field
-Proven effectiveness in identifying transition pathways in complex molecular systems, demonstrated through alanine dipeptide case study
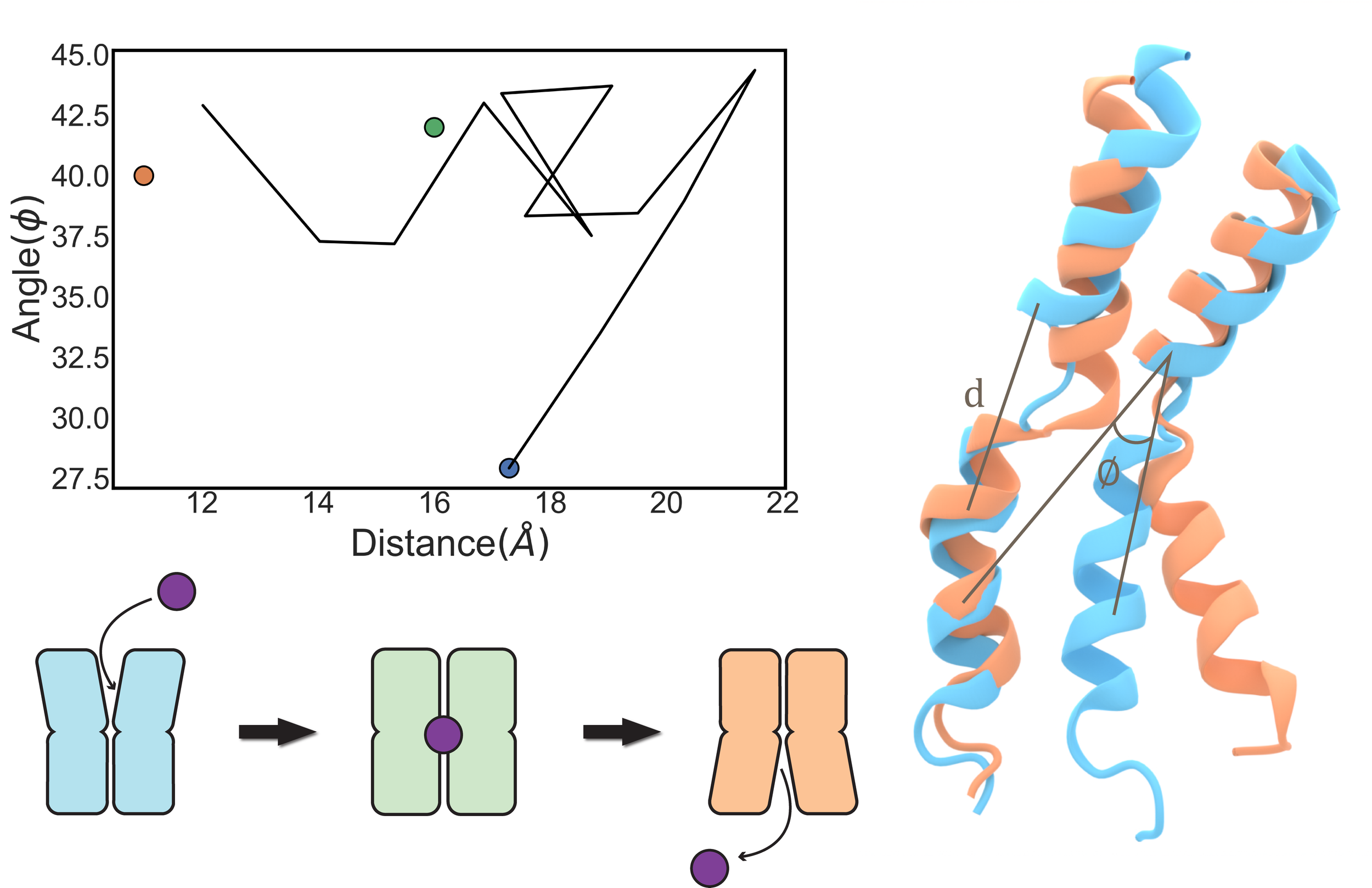